Understanding Bayesian Statistical Analysis in Business
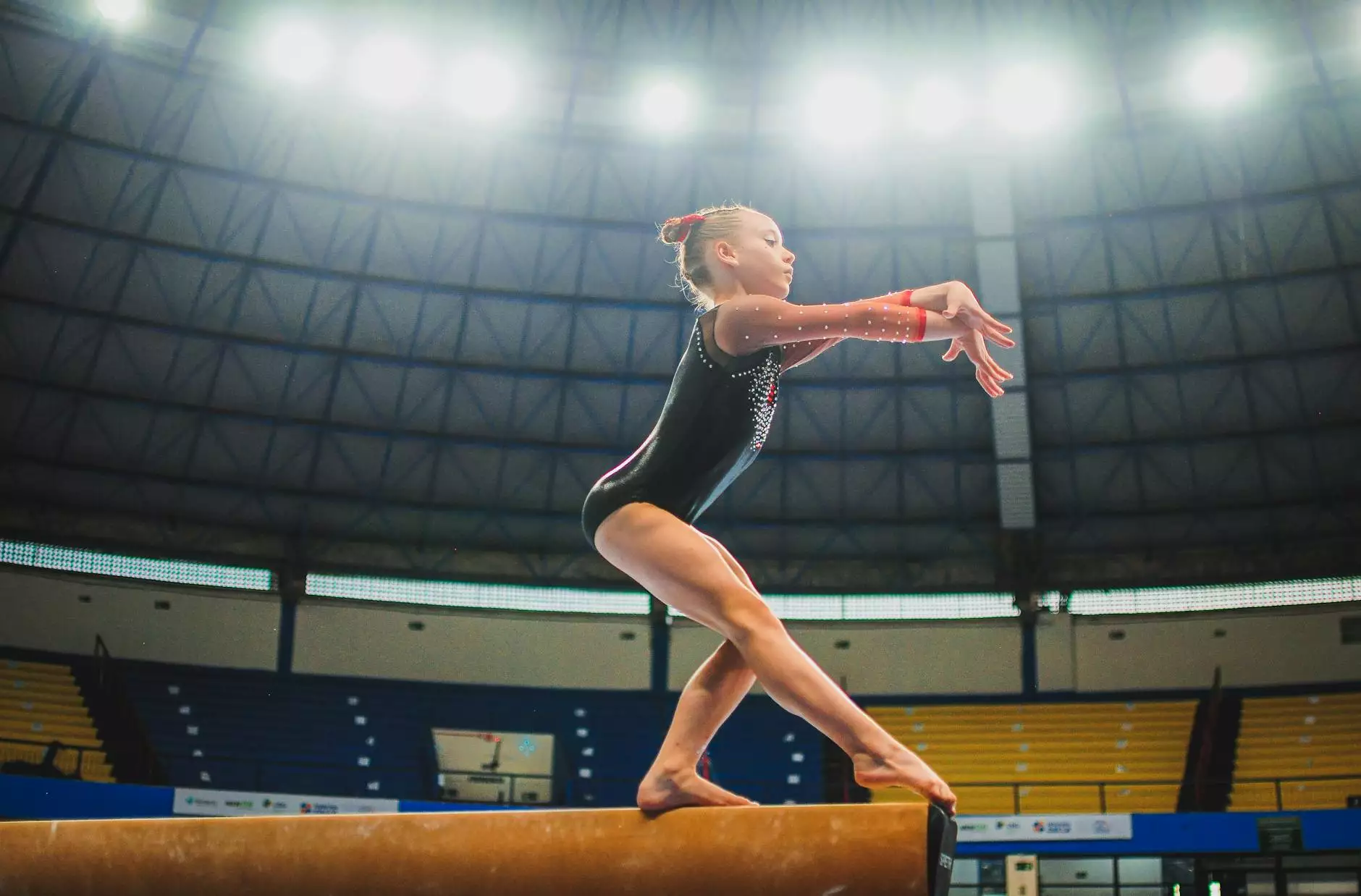
Bayesian statistical analysis is a powerful method that facilitates informed decision-making across various industries. This approach revolves around Bayes' theorem, which allows businesses to incorporate prior knowledge with new data to update their beliefs systematically. Whether in IT services, computer repair, or security systems, the insights gained through Bayesian analysis can provide a substantial competitive edge.
The Fundamentals of Bayesian Statistical Analysis
At its core, Bayesian statistical analysis is about updating probabilities based on new evidence. It provides a framework to quantify uncertainty and make better predictions. This methodology stands in contrast to traditional statistical methods, which often rely on fixed parameters and assumptions. Instead, Bayesian methods embrace the idea that knowledge is always evolving based on the information available.
Bayes' Theorem Explained
Bayes' theorem is the cornerstone of Bayesian analysis, given by the formula:
P(A|B) = (P(B|A) * P(A)) / P(B)Where:
- P(A|B) is the posterior probability (the probability of hypothesis A given the data B).
- P(B|A) is the likelihood (the probability of data B given hypothesis A).
- P(A) is the prior probability (the initial belief about hypothesis A before seeing data B).
- P(B) is the marginal likelihood (the total probability of data B under all hypotheses).
This theorem allows businesses to start with an initial belief, adjust it with new data, and continuously refine expectations about future outcomes. The dynamic adaptation inherent in Bayesian methods is what makes them unique and powerful for business applications.
The Role of Bayesian Analysis in Business Decision-Making
Businesses today face complex challenges requiring data-driven decision-making. Bayesian analysis plays a vital role in providing clarity amidst uncertainty. Below are several ways this methodology is applied in various business contexts:
1. Predictive Analytics
Predictive analytics aims to forecast future outcomes based on historical data. With Bayesian statistical analysis, businesses can develop models that not only predict likely outcomes but also quantify the uncertainty associated with these predictions. This enables organizations to proactively plan for various scenarios, making informed decisions that align with their strategic objectives.
2. Risk Management
Every business faces risks, from financial uncertainties to project failures. Bayesian analysis helps in assessing and managing these risks effectively. By calculating the probabilities of different risks and their potential impacts, businesses can allocate resources more efficiently and mitigate adverse outcomes.
3. Customer Insights and Personalization
In an era where personalized customer experiences are paramount, Bayesian methods facilitate deep insights into customer behavior. Businesses can segment customers based on evolving data patterns and tailor their marketing strategies accordingly. This not only enhances customer satisfaction but also improves conversion rates.
Applications of Bayesian Analysis in IT Services & Computer Repair
In fields such as IT services and computer repair, Bayesian statistical analysis has numerous applications:
1. Fault Prediction and Diagnosis
Bayesian models can be utilized to predict hardware failures or software malfunctions by analyzing historical data on system performance. For instance, by incorporating data on failure rates, maintenance logs, and user feedback, IT companies can forecast potential issues and address them before they escalate, minimizing downtime.
2. Optimizing Resource Allocation
For businesses offering computer repair services, knowing where to allocate resources effectively is crucial. Bayesian analysis aids in estimating the probability of service requests across different types of repairs, enabling companies to optimize staffing and inventory levels based on anticipated demand.
Impact of Bayesian Analysis in Security Systems
With increasing cybersecurity threats, Bayesian analysis becomes invaluable in developing robust security systems. Here’s how:
1. Threat Detection and Response
Bayesian methods enhance threat detection by analyzing patterns of user behavior and network activities. By establishing a baseline of normal behavior, systems can be trained to recognize anomalies that indicate potential security breaches. Furthermore, Bayesian approaches can prioritize responses based on the likelihood of threat severity.
2. Fraud Detection
Fraud detection is a critical aspect of security systems. Bayesian analysis allows organizations to incorporate prior knowledge about fraudulent activity in conjunction with current transaction data. This dual approach provides a probabilistic framework to assess the likelihood of fraud, helping to minimize false positives while effectively identifying genuine threats.
Advantages of Bayesian Statistical Analysis
Implementing Bayesian statistical analysis offers several advantages, particularly for businesses in IT services, computer repair, and security systems:
1. Incorporation of Prior Knowledge
One of the most significant benefits of Bayesian methods is their ability to incorporate prior knowledge or expert opinions into analysis. This feature allows businesses to use existing data or expert insights, enhancing the reliability of conclusions drawn from the analysis.
2. Flexibility and Adaptability
Bayesian models are inherently flexible. They can be adjusted as new information becomes available, making them suitable for dynamic environments characteristic of modern businesses. This adaptability is crucial for organizations that need to pivot quickly in response to changing market conditions.
3. Rich Interpretability
Bayesian analysis provides probability distributions for estimates, offering a richer interpretation of data than single-point estimates. This comprehensive view enables decision-makers to understand the uncertainties involved in their decisions better and act accordingly.
4. Facilitates Continuous Learning
With the ongoing influx of new data, businesses can continually refine their Bayesian models. Each update improves predictive accuracy, fostering a culture of continuous learning and enhancement within the organization.
Challenges of Bayesian Analysis and How to Overcome Them
While there are numerous advantages to Bayesian statistical analysis, businesses may encounter certain challenges during implementation:
1. Complexity of Implementation
The mathematical complexity and computational demands of Bayesian models can be a barrier to entry. To overcome this hurdle, organizations can invest in training their teams or collaborate with data scientists who specialize in Bayesian methods.
2. Subjectivity in Prior Selection
Selecting appropriate prior distributions can introduce subjectivity into the analysis. Businesses can address this by conducting sensitivity analyses to understand how different priors impact results, thereby ensuring that conclusions hold under various assumptions.
3. Computational Intensity
Bayesian methods often require significant computational resources, particularly for large datasets. Companies can leverage modern computational tools and cloud-based solutions to handle these demands efficiently, ensuring that they can scale their analytical efforts as needed.
The Future of Bayesian Statistical Analysis in Business
As businesses increasingly rely on data to drive their strategies, the demand for sophisticated analytical methods like Bayesian statistical analysis will continue to grow. The integration of machine learning and artificial intelligence with Bayesian methods holds immense potential for developing intelligent systems capable of making real-time decisions in complex environments.
1. Integration with Machine Learning
The combination of Bayesian analysis and machine learning creates powerful tools for predictive modeling. Bayesian models can be integrated into machine learning workflows to enhance model interpretability and uncertainty quantification, leading to more robust and flexible systems.
2. Real-time Data Processing
In an age where data is generated in real-time, the ability to process and analyze this information swiftly is crucial. Bayesian methods, especially through advancements in computational technologies, are positioned to handle real-time data effectively, allowing businesses to react promptly to new information.
Conclusion
In summary, Bayesian statistical analysis provides profound insights and advantages that can transform business decision-making. From predicting customer behavior to optimizing operations in IT services, computer repair, and security systems, this analytical approach is indispensable in navigating today’s complex business landscape. By embracing Bayesian methods, businesses can harness the power of data to inform their strategies, manage risks, and drive growth effectively.