Unlocking Opportunities with Agriculture Datasets for Machine Learning
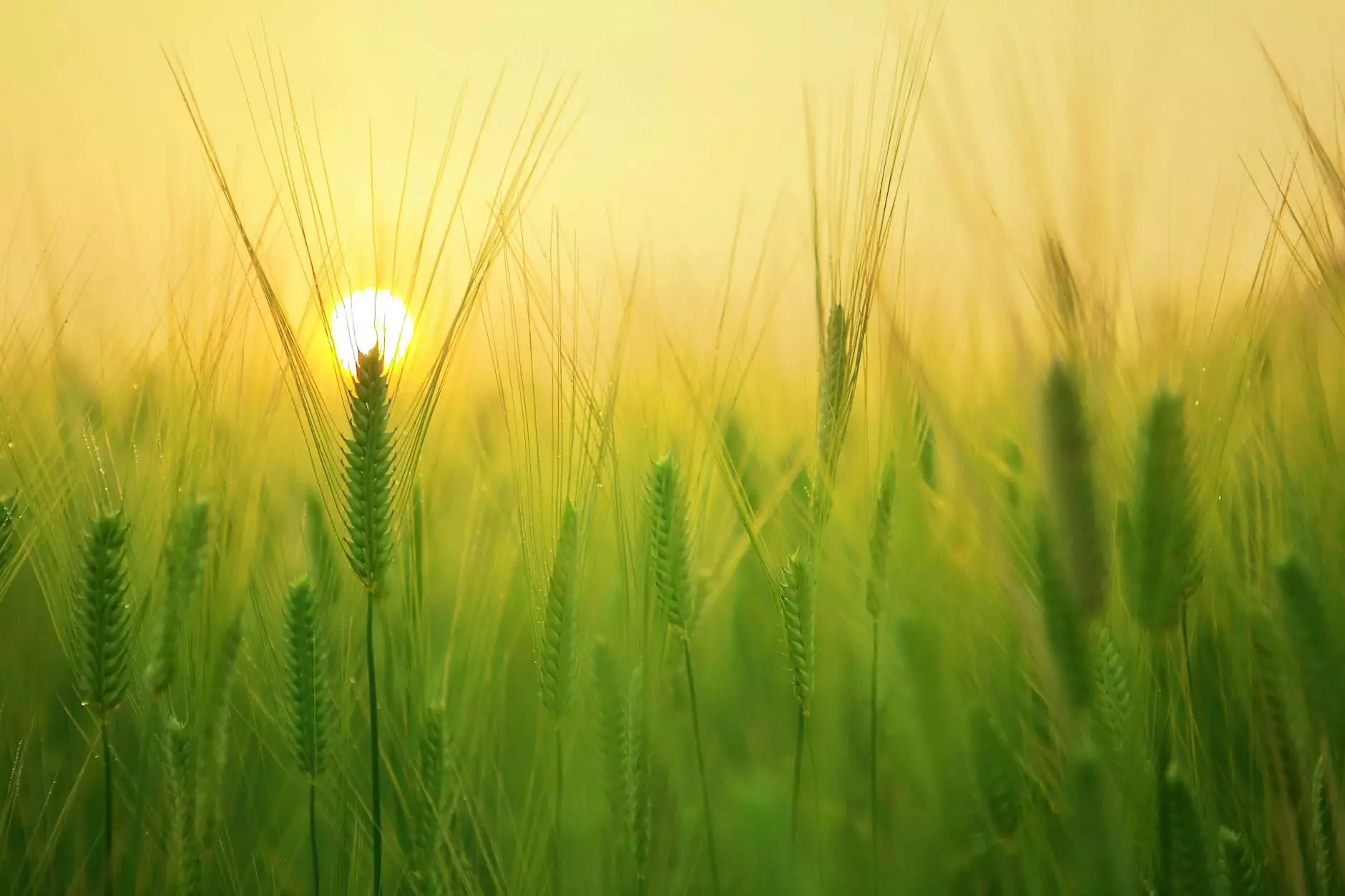
The agricultural sector is undergoing a significant transformation, and at the heart of this evolution is the integration of machine learning. By leveraging agriculture datasets for machine learning, farmers and agritech companies can harness data insights to unlock new levels of efficiency and sustainability. This article delves into the ways in which these datasets are revolutionizing agriculture, driving innovation, and addressing the challenges faced by the sector.
The Importance of Agriculture Datasets
In today's data-driven world, datasets play a crucial role, and agriculture is no exception. Agriculture datasets are collections of data related to various agricultural practices, crop yields, weather conditions, soil types, pest infestations, and livestock management. By utilizing these datasets, machine learning algorithms can process large volumes of information, enabling better predictive analytics and informed decision-making.
Types of Agriculture Datasets
Understanding the types of datasets available is essential for anyone looking to implement machine learning in agriculture. Here are some key categories of agriculture datasets:
- Crop Yield Data: Information on the yield of various crops under different conditions, helping to predict future harvests.
- Soil Data: Details about soil composition, moisture levels, and nutrient content, crucial for determining soil health.
- Weather Data: Historical and real-time data on weather patterns influencing agricultural practices.
- Pest and Disease Data: Information about pest outbreaks and plant diseases which can impact crop health.
- Market Price Data: Fluctuations in market prices for crops, aiding farmers in sales and financial planning.
Machine Learning Applications in Agriculture
Machine learning has a wide array of applications in agriculture, offering tools that enhance productivity and sustainability. Here, we explore some of the most impactful use cases:
1. Precision Agriculture
Precision agriculture leverages data to optimize field-level management regarding crop farming. By utilizing agriculture datasets for machine learning, farmers can:
- Monitor crop health through remote sensing technologies.
- Optimize irrigation systems based on real-time soil moisture data.
- Make informed decisions about fertilizer application to maximize yield while minimizing environmental impact.
This data-driven approach not only improves productivity but also promotes sustainable practices that are essential for the future of farming.
2. Predictive Analytics
Using machine learning algorithms, farmers can analyze historical data to forecast:
- Possible crop yields based on various factors.
- Market trends and pricing for better sales strategies.
- Pest outbreaks and disease patterns for preemptive action.
This ability to predict outcomes allows for proactive management rather than reactive measures, significantly improving operational efficiency.
3. Automated Farming Equipment
The advent of autonomous tractors and drones equipped with cameras and sensors is transforming farming practices. These tools can collect valuable data, which is then analyzed using machine learning to:
- Enhance planting techniques.
- Monitor field conditions in real-time.
- Optimize harvesting processes.
Automation, combined with agriculture datasets analysis, leads to higher yields and reduced labor costs.
4. Supply Chain Optimization
By integrating machine learning with agriculture datasets, supply chains can be optimized for better efficiency. Analysis of data helps in:
- Forecasting demand to prevent overproduction or shortages.
- Identifying the best transportation routes to reduce costs and time.
- Improving inventory management to minimize waste.
These optimizations ultimately lead to a more resilient agricultural sector that can respond swiftly to changes in demand and supply.
Case Studies: Success Stories in Agriculture and Machine Learning
Several agritech companies and farms are harnessing the power of agriculture datasets for machine learning to achieve remarkable success. Here are a few notable case studies:
Case Study 1: John Deere's Precision Solutions
John Deere, a leader in agricultural machinery, has integrated machine learning into its precision farming solutions. By using data collected from their advanced machinery, they offer farmers insights that lead to better planting decisions. Their machine learning algorithms analyze data from over 60 million acres to improve and predict seed efficacy, leading to staggering increases in yield.
Case Study 2: Taranis and Aerial Imagery
Taranis, an agritech startup, employs aerial imagery and machine learning to monitor crop health. Their platform analyzes images captured by drones to detect diseases or pests early. By processing agriculture datasets efficiently, they help farmers make timely interventions, significantly cutting down losses.
Case Study 3: IBM's Watson Decision Platform for Agriculture
IBM has harnessed its Watson AI to create a decision platform specifically for agriculture. By integrating weather data, soil information, and other datasets, farmers can receive actionable insights through advanced analytics, ultimately enhancing productivity and sustainability.
Challenges and Considerations
While the potential of machine learning in agriculture is immense, there are challenges that stakeholders must navigate:
- Data Quality: Ensuring high-quality, reliable data is crucial for effective machine learning outcomes.
- Data Privacy: As data collection rises, protecting sensitive information becomes increasingly important.
- Technical Skill Gap: Many farmers may lack the technical background needed to utilize complex machine learning tools.
- Cost of Implementation: The upfront costs associated with adopting new technologies can be a barrier for some farmers.
Addressing these challenges through education, investment in technology, and supportive policies is vital for the widespread adoption of machine learning in agriculture.
Future Trends in Agriculture and Machine Learning
As technology evolves, so too will the role of machine learning in agriculture. Several trends are poised to shape the future:
- Integration of IoT Devices: The Internet of Things (IoT) will enable even more extensive data collection from farms, providing richer datasets for machine learning analysis.
- Increased Focus on Sustainability: Machine learning will play a crucial role in promoting sustainable practices that are essential as the world confronts climate change.
- Collaborative Platforms: Enhanced data sharing among farmers, research institutions, and corporations can lead to innovative solutions and collective problem-solving.
- AI-Powered Decision Making: Advanced AI algorithms will provide real-time, actionable insights, transforming how farmers operate.
Conclusion
The integration of agriculture datasets for machine learning represents a significant step forward for the agricultural industry. Through strategic application, farmers can increase efficiency, enhance sustainability, and make informed decisions that drive productivity. By remaining aware of the challenges and embracing future trends, the agricultural sector can continue to innovate and thrive in an increasingly competitive landscape.
As the industry evolves, those who harness the power of data and machine learning will lead the way in shaping the future of agriculture. Whether you are a farmer, technologist, or stakeholder, embracing this revolution is essential for achieving sustainable success in agriculture.
agriculture dataset for machine learning