Revolutionizing Video Labeling with Machine Learning
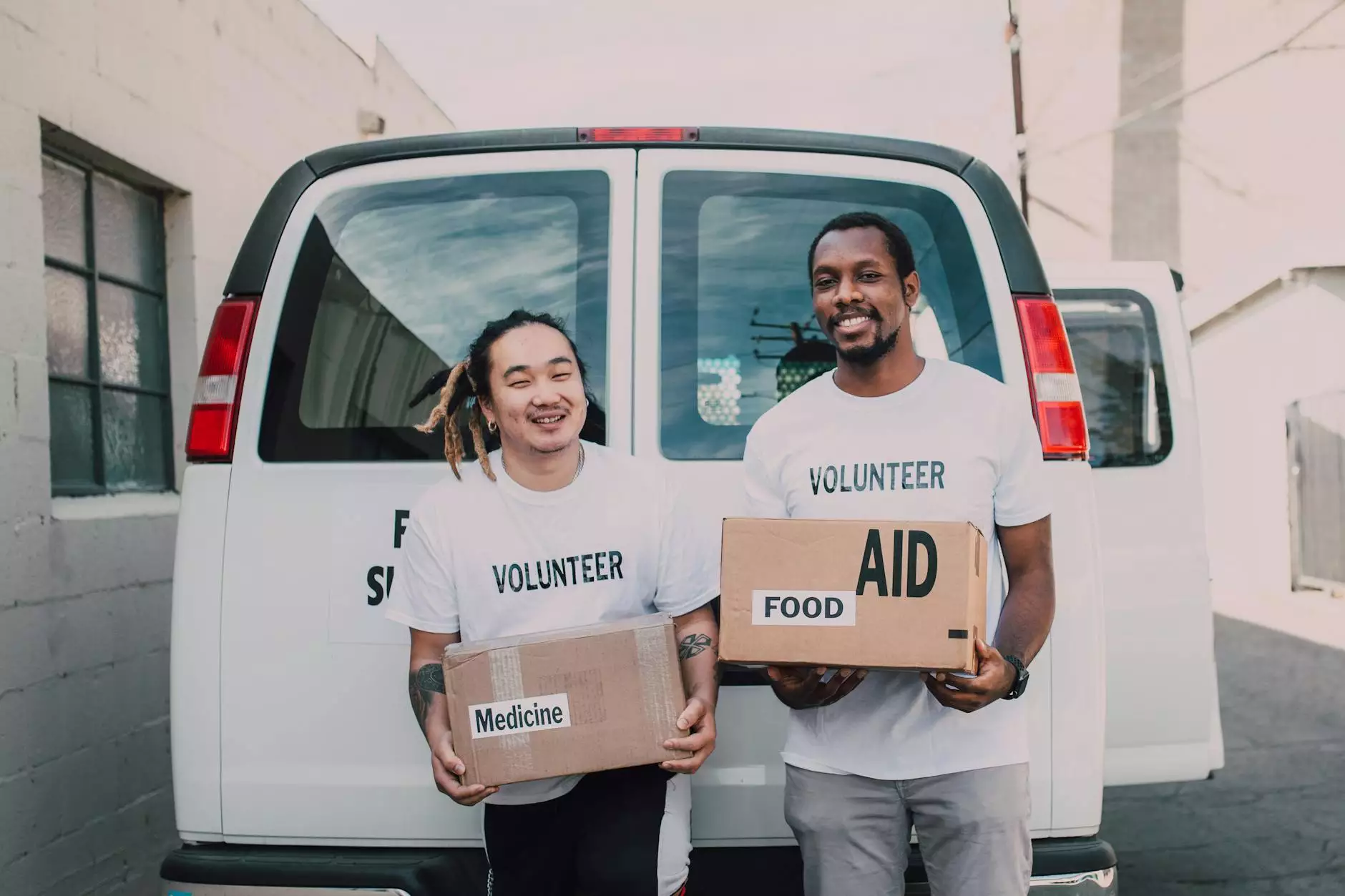
In today’s digital landscape, the demand for effective data annotation tools is greater than ever. As businesses across various sectors seek to harness the power of artificial intelligence (AI), machine learning has emerged as a pivotal element in enhancing the capabilities of video labeling tools. A well-executed video labeling tool powered by machine learning not only elevates the accuracy of annotations but also streamlines the workflow, allowing companies to maximize their productivity.
Understanding Video Labeling Tools
Video labeling tools facilitate the process of assigning meaningful annotations to videos, a crucial step for training machine learning models. These annotations may range from identifying objects in a scene to outlining specific actions occurring within the video. The accuracy of these annotations directly impacts the model's performance, which is why an efficient labeling tool is fundamental.
What Makes Video Labeling Essential?
- Data Quality: High-quality annotated data is vital for the success of machine learning projects.
- Scalability: As video content multiplies, the need for scalable solutions in video labeling grows.
- Automated Processes: Automation significantly reduces the time required for data preparation.
- Improved Accuracy: Enhanced labeling tools reduce human error, ensuring comprehensive data sets.
The Role of Machine Learning in Video Annotation
Machine learning plays a transformative role in the development of sophisticated video labeling tools. By integrating algorithms that can learn from data, these tools can automate much of the annotation process. This results in a crisp, efficient workflow and drastically reduces the time and labor costs associated with traditional, manual labeling methods.
How Machine Learning Enhances Video Labeling
The following are some key advantages of incorporating machine learning into video labeling tools:
- Automatic Object Recognition: Machine learning algorithms can be trained to identify and classify objects within video frames, significantly speeding up the labeling process.
- Context Understanding: Advanced models can understand context and infer the relationships between objects, allowing for more nuanced annotations.
- Adaptive Learning: Adjusting to new data types, machine learning models can continually improve their accuracy over time based on iterative learning processes.
- Reduced Human Oversight: By automating repetitive tasks, human annotators can focus on more complex aspects of video analysis, ensuring better outcomes.
Choosing the Right Video Labeling Tool
The market is flooded with video labeling tools, each with varying features and capabilities. Choosing the right tool can significantly influence the efficiency and effectiveness of your data annotation process. Here are some critical factors to consider when selecting a video labeling tool:
Critical Features to Look For
- User-Friendly Interface: A tool that is easy to navigate can drastically reduce the learning curve for new users.
- Flexible Annotation Options: Ensure the tool supports various types of annotations, such as bounding boxes, polygons, and semantic segmentation.
- Integration Capabilities: The tool should seamlessly integrate with other platforms in your workflow, enhancing overall productivity.
- Support for Machine Learning Frameworks: Choose a tool that can export data in formats compatible with popular machine learning frameworks like TensorFlow, PyTorch, and others.
- Collaboration Features: Look for tools that allow multiple users to collaborate on projects, facilitating better communication and efficiency.
The Future of Video Labeling in the Era of AI
As businesses continue to evolve, the importance of video labeling tools will only grow. Innovations in machine learning are paving the way for smarter, more autonomous labeling solutions that save time and resources. Companies like KeyLabs AI are at the forefront of this transformation, providing state-of-the-art data annotation tools designed to meet the evolving needs of various industries.
Emerging Trends in Video Labeling
The following emerging trends signify the future direction of video labeling tools:
- Deep Learning Integration: Deep learning methodologies are expected to integrate more deeply with video annotation tools, enhancing their ability to understand and interpret complex visual data.
- Real-Time Labeling: Advances in processing capabilities will allow for real-time labeling of video streams, making it invaluable for applications in surveillance, sports analytics, and live event coverage.
- Enhanced User Training: As machine learning evolves, there will be more emphasis on training users to leverage these tools effectively, maximizing their potential.
- Semantic Segmentation Capabilities: Tools will increasingly focus on providing segmented annotations that enable a clearer understanding of scenes and interactions.
Conclusion
In conclusion, the advent of machine learning has revolutionized the realm of video labeling tools, offering unprecedented efficiency and accuracy in data annotation. The future looks bright as advancements continue to be made, paving the way for smarter, more capable solutions. Businesses investing in these technologies will undeniably enjoy a competitive advantage, streamlining their operations and harnessing the full power of their video data.
By choosing tools from KeyLabs AI, organizations can ensure that they are utilizing the latest innovations in data annotation, propelling them towards success in their respective industries.
video labeling tool machine learning